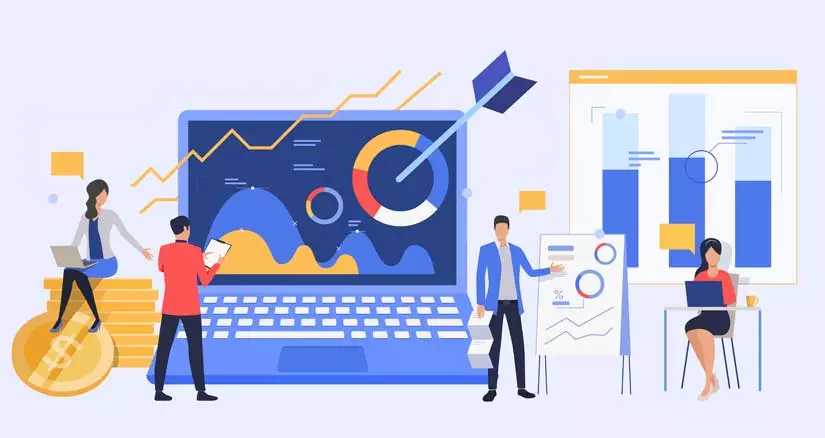
Enquire Now
Duration : 6 Months / Mode : Part Time
ABOUT [DATA ANALYTICS]
Data analytics is the process of examining large datasets to uncover meaningful insights, trends, and patterns that can inform decision-making and drive business outcomes. It involves collecting, cleaning, transforming, and analyzing data from various sources to extract actionable insights and valuable information. Here’s an overview of data analytics and its key aspects:
Data Collection and Integration: Data analytics begins with collecting data from diverse sources, including databases, spreadsheets, websites, sensors, social media platforms, and IoT devices. Data may be structured (e.g., databases, spreadsheets) or unstructured (e.g., text, images, videos). Once collected, data needs to be integrated and stored in a centralized location for analysis.
Data Cleaning and Preparation: Before analysis, data often requires cleaning and preparation to ensure accuracy, consistency, and completeness. This involves removing duplicates, handling missing values, standardizing formats, and transforming data into a usable format for analysis. Data cleaning is essential for improving the quality and reliability of insights derived from analytics.
Data Exploration and Visualization: Data analytics involves exploring and visualizing data to gain a deeper understanding of patterns, relationships, and trends. Visualization techniques such as charts, graphs, heatmaps, and dashboards help identify patterns, outliers, correlations, and insights that may not be apparent in raw data. Visualization enhances data comprehension and facilitates communication of findings to stakeholders.
Descriptive Analytics: Descriptive analytics focuses on summarizing historical data to describe what has happened in the past. It involves generating summary statistics, frequency distributions, and visualizations to understand the current state of affairs and identify trends or patterns in the data.
Diagnostic Analytics: Diagnostic analytics aims to understand why certain events occurred by investigating causal relationships and identifying factors that contribute to specific outcomes or trends. It involves conducting root cause analysis, hypothesis testing, and correlation analysis to uncover insights into the underlying causes of observed patterns.
Predictive Analytics: Predictive analytics leverages statistical modeling, machine learning algorithms, and data mining techniques to forecast future outcomes or trends based on historical data. It involves building predictive models, such as regression, classification, clustering, and time series forecasting, to make predictions and inform decision-making.
Prescriptive Analytics: Prescriptive analytics goes beyond predicting future outcomes to recommend actions or strategies to achieve desired outcomes. It involves optimizing decision-making by simulating different scenarios, evaluating potential actions, and identifying the most effective course of action based on data-driven insights.
Data Governance and Security: Data analytics requires robust data governance practices to ensure data quality, integrity, privacy, and security. This includes establishing data policies, standards, and procedures, implementing access controls, encryption, and data anonymization techniques, and complying with regulatory requirements (e.g., GDPR, HIPAA).
Tools and Technologies: Data analytics relies on a variety of tools and technologies to collect, store, process, analyze, and visualize data. This includes data analytics platforms (e.g., Python, R, SQL), data visualization tools (e.g., Tableau, Power BI), and cloud-based analytics services (e.g., AWS, Google Cloud, Azure).
Overall, data analytics plays a crucial role in unlocking the value of data by transforming raw data into actionable insights, enabling organizations to make data-driven decisions, optimize processes, and gain a competitive advantage in today’s data-driven business landscape.